Unveiling the Mysteries of AI: A Deep Dive into 2023's Landmark XAI Paper in Information Fusion
- vazquezgz
- May 1, 2024
- 4 min read
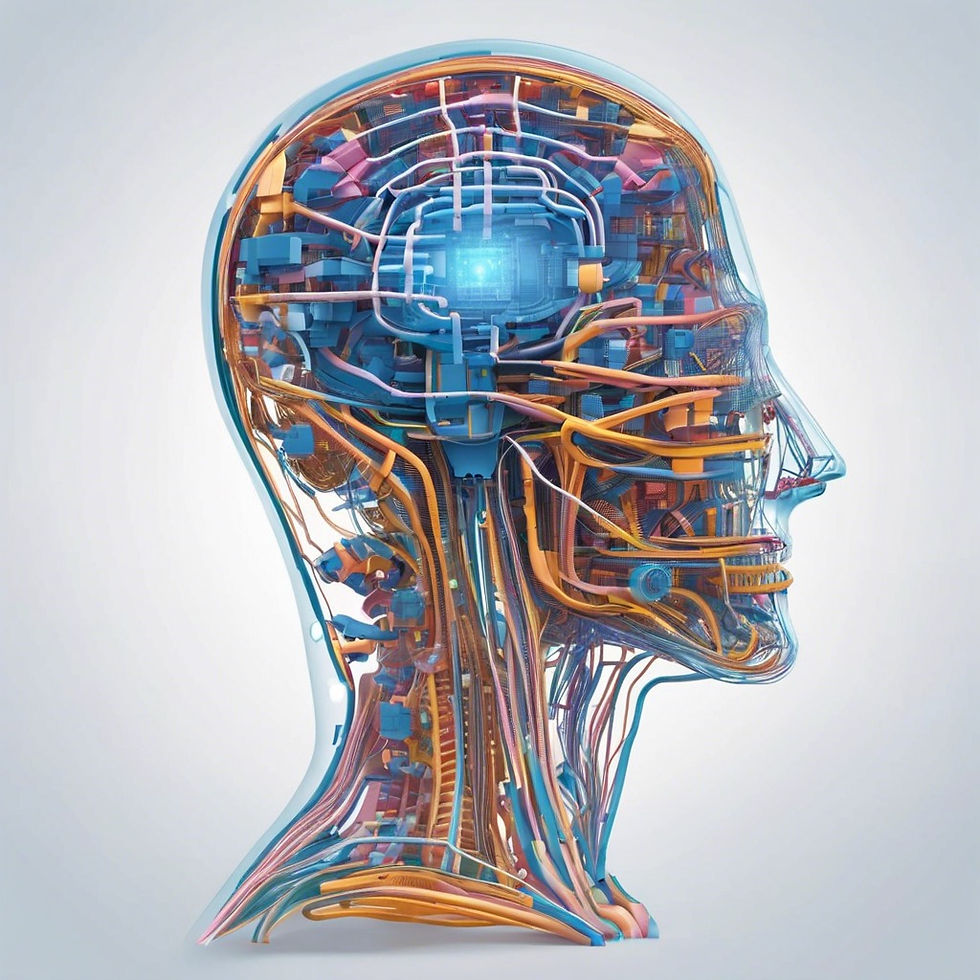
In the evolving field of Explainable Artificial Intelligence (XAI), a significant contribution was made in 2023 with a comprehensive study published in the journal Information Fusion. This paper was authored by a distinguished group of researchers from several prestigious institutions. Leading the study from Sungkyunkwan University's Information Laboratory (InfoLab) were Sajid Ali, Tamer Abuhmed, and Shaker El-Sappagh, who also holds affiliations with Galala University and Benha University in Egypt. They were joined by Khan Muhammad from the same university's Visual Analytics for Knowledge Laboratory (VIS2KNOW Lab), highlighting a strong representation from South Korea.
The European contribution to the research team was robust, with Jose M. Alonso-Moral from Centro Singular de Investigación en Tecnoloxías Intelixentes (CiTIUS) at Universidade de Santiago de Compostela in Spain, Roberto Confalonieri from the University of Padua, Italy, and Riccardo Guidotti from the University of Pisa, Italy. Javier Del Ser brought expertise from TECNALIA and the Basque Research and Technology Alliance (BRTA), Spain, while Natalia Díaz-Rodríguez and Francisco Herrera from the University of Granada’s Andalusian Research Institute in Data Science and Computational Intelligence (DaSCI) completed the team, ensuring a diverse and comprehensive exploration of XAI from a global perspective.
This comprehensive review stands out as a valuable reference for anyone interested in Explainable AI (XAI) because it not only highlights the technical nuances of the methodologies but also skillfully contextualizes their importance within the broader AI ecosystem. This dual approach helps to bridge the gap between complex technical details and their practical implications, making it an essential resource for both researchers and practitioners aiming to understand and apply XAI principles effectively.
Inside the Paper
The paper, titled "Advanced Methods for Knowledge Extraction in Machine Learning: An Information Fusion Perspective," serves as a critical resource for anyone interested in the inner workings of complex AI systems. The authors have meticulously categorized knowledge extraction techniques into three primary types: decompositional, pedagogical, and eclectic methods. Each category is explored with depth and clarity, providing insights into how these methods can unveil the mysterious decision-making processes of ANNs.
Rule Extraction Techniques
A significant portion of the paper is dedicated to rule extraction techniques. These are methods that simplify the outputs of machine learning models into rules that are easier for humans to understand, such as IF-THEN statements. The authors discuss various forms of these techniques, from the simplest conditional rules to more complex Boolean and fuzzy logic systems. This discussion is particularly valuable for those looking to implement practical AI applications where understanding the basis of model decisions is crucial.
Understandability and Satisfaction
The paper doesn't just stop at extraction techniques; it also addresses the impact of these methods on the end-user. How do these extracted rules and knowledge pieces affect user satisfaction and trust in AI systems? The researchers present findings from surveys and interviews that measure user reactions to different explanation formats. This aspect is vital as it underscores the need for explainability not just from a technical standpoint but also from a user-experience perspective.
Trust and Transparency
Trust is a central theme of the paper. The authors argue that transparency in AI operations fosters trust among users, which is essential for the widespread adoption of AI technologies. They explore various frameworks and tools designed to enhance the transparency of AI systems, assessing their effectiveness through empirical studies. This section is particularly insightful for developers and stakeholders in AI who are working towards building more reliable and ethical systems.
Computational Assessment of Explanations
Another groundbreaking aspect discussed is the computational assessment of explanations provided by AI systems. The paper critiques the reliance on human judgment alone to validate AI explanations and advocates for computational methods that can objectively assess the fidelity and accuracy of these explanations. This approach promises a more rigorous verification of AI explainability, ensuring that systems perform as intended and are free from biases or errors that could mislead users.
Where to Find the Paper
For those eager to dive into the detailed discussions and analyses, the paper is available in the April 2023 issue of Information Fusion, Explainable Artificial Intelligence (XAI): What we know and what is left to attain Trustworthy Artificial Intelligence - ScienceDirect. This paper is a must-read for anyone involved in AI development, from researchers to practitioners, as it provides both a deep theoretical foundation and practical insights into the implementation of explainable AI.
Conclusion: Setting New Standards in AI Transparency and Trust
The insightful analysis provided in the April 2023 issue of Information Fusion represents a significant milestone in the field of explainable artificial intelligence (XAI). This paper not only deepens our understanding of the mechanisms behind AI decision-making but also pioneers the bridge between complex AI behaviors and human interpretability. It underscores the urgency and necessity of integrating explainability at the core of AI system development, reinforcing that the future of AI should be as much about clarity and openness as it is about sophistication and efficiency.
This work is a compelling reminder that the true power of AI lies not only in its computational capabilities but also in its accessibility to the people who use it. The emphasis on making AI systems transparent and trustworthy is crucial for securing user trust—a key ingredient for the broader acceptance and ethical integration of AI technologies in society. By pushing the boundaries of how we understand and interact with AI, this paper sets a new benchmark for what it means to build not just more intelligent, but also more inclusive and accountable, AI systems.
Looking ahead, the path forward for AI involves a dual commitment to advancing technological frontiers while enhancing the explainability of these systems. The approaches and insights presented in this research not only guide current practitioners but also serve as a foundational stone for future explorations in XAI. They prompt us to envision a world where AI systems are partners in human progress, understood and trusted by all users. This paper, thus, is not just a scholarly article; it is a manifesto for future AI practices—where transparency, user satisfaction, and trust go hand in hand with technological advancement.
As AI continues to weave itself into the fabric of daily life, let us ensure that its growth is complemented by an equally robust development in our ability to explain, understand, and responsibly deploy it. The journey towards truly trustworthy AI is complex and continuous, and contributions like those detailed in this paper are vital beacons along this path.
Comments